How To Become a Data Scientist
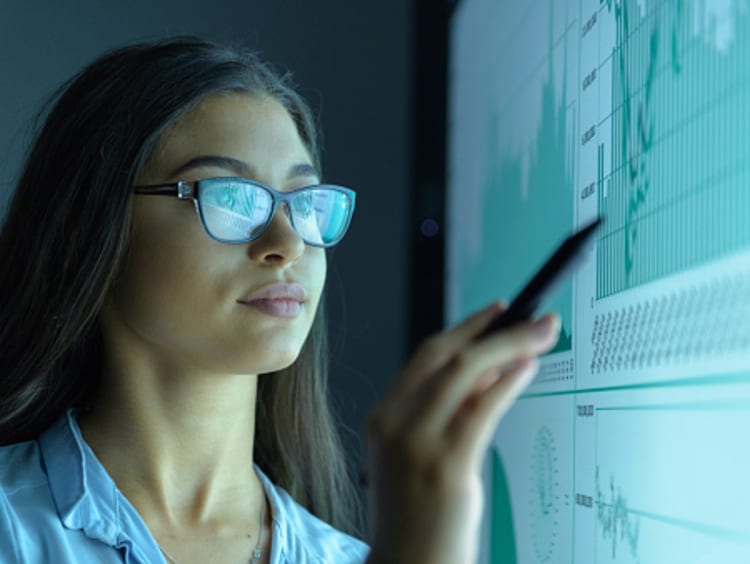
Students who have a knack for numbers, a passion for technology and a desire to help organizations solve complex real-world problems might consider pursuing a career in data analytics. This burgeoning field has implications for all sorts of industries, from technology to national security. Explore this career guide to learn what data scientists do and what is the pathway for how to become a data scientist.
What Does a Data Scientist Do?
The job of a data scientist depends largely on the type of organization they work for. These professionals must first thoroughly understand the objectives of their organization and/or department, and then assess how big data analytics can be used to achieve those goals. The specific tasks of a data scientist can include any of the following:
- Design data modeling processes
- Develop algorithms and predictive models
- Process the data and “clean” it to extract the relevant data
- Conduct a data analysis using data science techniques like machine learning, statistical modeling and artificial intelligence
- Evaluate the results and present insights to organizational stakeholders
Which Industries Hire Data Scientists?
Virtually every industry can benefit from the insights provided by data scientists. Some of the key industries that hire data scientists are as follows:
- Retail – Every type of retail business stakes its success on anticipating what customers will need and want, and then ordering inventory based on those trends. Data analytics is integral to developing accurate insights about consumer trends. Some retailers even use data science to develop highly personalized shopping experiences for their customers.
- Healthcare – Healthcare managers are always on the lookout for ways of making healthcare delivery more efficient, better for the patients and less costly. Data scientists can study medical data to make suggestions for improvements.
- Finance and banking – The banking industry is increasingly using technology to connect with customers and inform decision making.
- Transportation – Data scientists working for the transportation industry might do anything from assess how to reduce traffic jams to how to improve the customer experience on public transportation modes.
These are just a few examples. Construction, education, manufacturing, energy and natural resource extraction are other industries in which big data plays an increasingly important role.
Lastly, some data scientists work for government agencies. For instance, the U.S. Food and Drug Administration (FDA) can use data science techniques to evaluate patterns regarding the outbreaks of food-borne illnesses.
An Overview of Data Scientists
High school students who are interested in pursuing the data scientist career path are advised to speak with their guidance counselor about their career goals. It may be possible to adjust one’s schedule to better prepare for the future, such as by adding more mathematics classes. Courses in computer science, particularly software applications will also be helpful.
As the high school graduation date approaches, students will need to plan on earning at least a bachelor’s degree. It’s ideal to earn a bachelor’s degree in computer science with a concentration in data analytics or big data, although there is some flexibility in this field. Also, for students who are more business-oriented, a bachelor’s degree in business analytics is also an option. Following these four-year degree programs, graduates are strongly encouraged to consider pursuing a master’s degree and/or a doctoral degree, as these advanced qualifications can open up greater job prospects.
Earning an Undergraduate Data Analytics Degree
After high school, the first step toward pursuing a career in data science is to earn a relevant undergraduate degree. There is no universal requirement regarding the exact type of degree a student should earn. However, data science, computer science, or business analytics degrees are generally preferred by employers. Consider looking for a Bachelor of Science in Computer Science with an Emphasis in Big Data Analytics degree.
Students in data science degree programs will study how to use existing data science methodologies, techniques and algorithms. They’ll also learn how to develop their own algorithms. Some of the specific topics students may study can include the following:
- The modern theories, design and implementation models for large scale information systems
- Discrete mathematics with digital logic, including Boolean algebra
- Computer architecture, including performance measurement, memory hierarchies and multiprocessors
- The development of algorithms for organizing and searching very large data sets
- Differential equations and their use in modeling and simulation
Students in business analytics degree programs will focus less on theory and more on practical application of data science techniques using industry software tools. The Bachelor of Science (BS) Business Analytics degree is one such degree program.
Pursuing a Master’s Degree in Data Analytics
It’s possible to land an entry-level job in data science with a bachelor’s degree. However, graduates who are new to the field are likely to find that many jobs in data science do require a master’s degree. Even if a master’s degree isn’t a strict requirement, employers often prefer to hire data scientists who hold one.
A master’s degree, like an MS in Data Science, is also a must-have for aspiring data scientists who earned a bachelor’s degree that wasn’t specifically focused on data science. Individuals may decide to immediately move on to graduate school after earning a bachelor’s degree. Alternatively, they might choose to pursue entry-level employment while working toward a degree in their spare time.
The average master’s degree takes two years of full-time study to complete. Those who choose to work while attending school on a part-time basis may need about three years to complete their degree. A master’s degree program will take a deep dive into the critical topics that aspiring data scientists need to know, such as the following:
- Foundational principles in predictive modeling
- Methods and applications of linear regression and multivariate analysis
- The development and use of data mining algorithms
- The design and creation of software applications
Earning a Doctorate in Data Analytics
After gaining at least a few years of experience in the field, data scientists may decide they’re ready to head back to school to pursue a PhD. Although earning a doctoral data analytics degree is not strictly necessary for data scientists, doing so can be hugely beneficial.
Working toward a PhD requires more than a few years of intensive research and experimentation. PhD candidates may test new methodologies and experiment with new data science technologies. This allows them to work on the cutting-edge of emerging developments in the field, gaining the advanced skills and in-depth knowledge that employers value.
After completing and successfully defending their dissertation in data science, PhD graduates can typically enjoy the following benefits:
- Stronger qualifications to pursue senior-level positions
- Better potential to command a top salary
- Advanced knowledge and skills that make professionals more effective at their work
- Professional respect and recognition for their expertise
At Grand Canyon University, you can begin building a solid academic foundation from which to pursue a career as a data scientist. In addition to the many undergraduate degree programs, STEM students at GCU can choose from graduate programs such as the Master of Science in Data Science degree and the Doctor of Business Administration: Data Analytics (Quantitative Research) program. Click on Request Info at the top of your screen to begin exploring your future at GCU.
The views and opinions expressed in this article are those of the author’s and do not necessarily reflect the official policy or position of Grand Canyon University. Any sources cited were accurate as of the publish date.